A statistical exploration of interval-deficient wind speed data for application to wind power assessments
DOI:
https://doi.org/10.17159/2413-3051/2019/v30i4a5694Keywords:
wind power assessment, renewable resource modelling, Weibull distribution, interval deficient data, sauran, feasibility study cost reductionAbstract
Gathering quality wind speed data can be time-consuming and expensive. The present study established whether interval-deficient wind speed data could be rendered useful for wind power assessments. The effect of interval deficiency on the quality of the wind speed data was investigated by studying the behaviour of the Weibull scale and shape factors as the interval size between wind speed measurements increased. Five wind speed data sets obtained from the Southern African Universities Radiometric Network (Sauran) were analysed, based on a proposed procedure to find the true Weibull parameters from an interval-deficient wind speed data set. It was found that the relative errors in the Weibull parameters were, on average, less than 1%, compared with the Weibull parameters computed from a wind speed data set that complies with the IEC 61400-12-1:2005(E) standard. This finding may contribute to time and cost reduction in wind power assessments. It may also promote the application of statistical methods in the renewable energy sector.
Downloads
Metrics
References
Abernethy, R.B. (1983). Weibull Analysis Handbook. West Palm Beach, Florida.
Brooks, M.J., du Clou, S., van Niekerk, J.L., Gauche, P., Leonard, C., Mouzouris, M.J., Meyer, A.J., van der Westhuizen, N., van Dyk, E. and Vorster, F.J. (2015). SAURAN: A new resource for solar radiometric data in Southern Africa. Journal of Energy in Southern Africa, vol. 26, no. 1, pp. 2–10. ISSN 1021447X.
Drobinski, P. and Coulais, C. (2012). Is the Weibull distribution really suited for wind statistics modelling and wind power evaluation? arXiv:1211.3853v1.
Justus, C.G., Hargraves, W.R. and Yalcin, A. (1976). Nationwide assessment of potential output from wind-powered generators. Available at: http://journals.ametsoc.org/doi/abs/10.1175/1520-0450(1976)015{%}3C0673: NAOPOF{%}3E2.0.CO;2
Ramirez, P. and Carta, J.A. (2005). Influence of the data sampling interval in the estimation of the parameters of the Weibull wind speed probability density distribution: a case study. Energy Conservation and Management, vol. 46, pp. 2420–2438.
Sauran (2017). South African Universities Radiometric Network. Available at: www.sauran.net
Scholz, F. (2008). Inference for the Weibull Distribution. vol. 632.
Seguro, J.V. and Lambert, T.W. (2000). Modern estimation of the parameters of the Weibull wind speed distribution for wind energy analysis. Journal of Wind Engineering and Industrial Aerodynamics, vol. 85, no. 1, pp. 75–84. ISSN 01676105.
Siepker, E.B.J. and Harms, T.M. (2017). On the potential value of interval deficient wind data. Journal of Wind Engineering and Industrial Aerodynamics, vol. 165, no. February, pp. 100–114. ISSN 01676105. Available at: http://linkinghub.elsevier.com/retrieve/pii/S0167610515302294
Underhill, L. and Bradfield, D. (2013). Les Underhill and Dave Bradfield. , no. January.
Walpole, R.E., Myers, R.H., Myers, S.L. and Ye, K. (2016). Probability & Statistics for Engineers and Scientists. Pearson.
Weibull, W. (1951). A Statistical Distribution of Wide Applicability. Journal of Applied Mechanics, pp. 293–297.
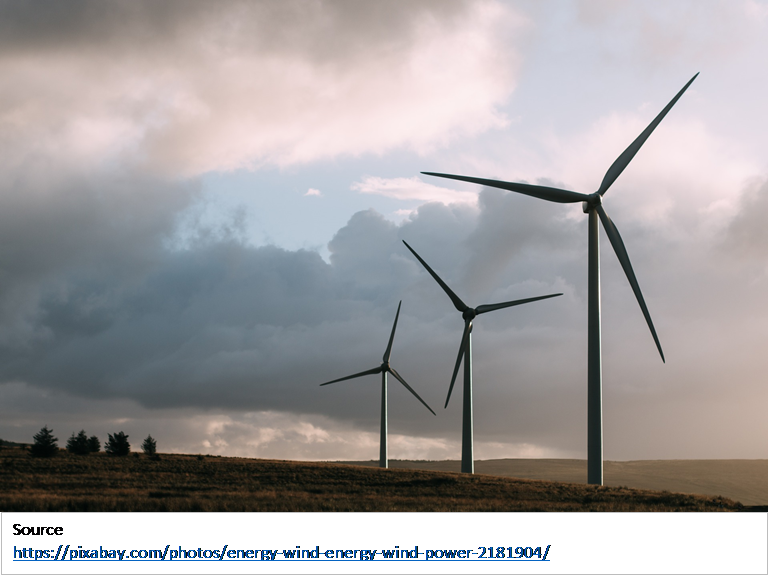
Downloads
Published
How to Cite
Issue
Section
License
Copyright (c) 2019 F. Lubbe, T. Harms, J. Maritz

This work is licensed under a Creative Commons Attribution-ShareAlike 4.0 International License.